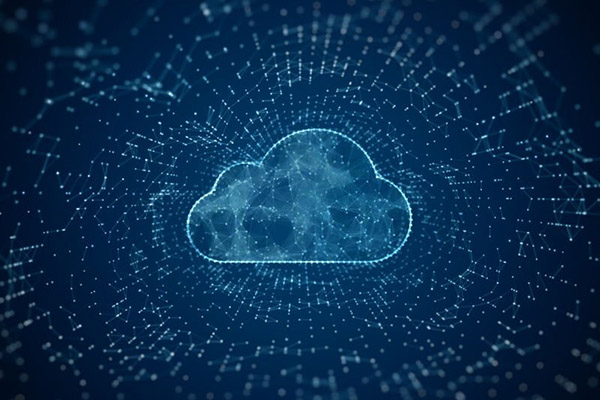
Data Gravity, the Source Cooperative and hopeful thoughts...
TL;DR
- understand the cost drivers in your "open data" strategy, long-term
- don't neglect the ecosystem gravitating around the actual data
- you can outsource data promotion but not data governance
- allow a neutral cooperative to track uptake and share analytics
The term data gravity (opens new window) describes the observation that data has mass and once it reaches a certain volume, even more data - but also applications and services - are attracted to its location. In the world of Earth Observation (EO) and Machine Learning (ML) that mass may grow quite fast and the greater its gravitational pull, the more difficult (and more expensive) it becomes to move later on. This gravitational pull with its tendency for centralization is not necessarily a bad thing. Having all data, applications and services at one location makes finding and exploiting the data potentially easier. And if we are talking about a public (AWS, Azure, Google, etc.) or a domain specific cloud (like the Copernicus Data Space Ecosystem - CDSE) as data location, the elastic compute close to the data storage layer may enable large-scale workflows and efficient collaboration.
Cloud commoditization opened up new possibilities to drive or contribute to open science initiatives, with companies like EOX trying to streamline the processes for scientists to produce new (usually "open") data on one hand and making these data set widely (or even publicly) accessible on the other hand. In this context governmental institutions or agencies like the European Space Agency (ESA) take the mandate to not just act as provider of "open data" by covering the costs of production and maintenance, but also to foster the uptake of these data sets and contribute to the good of society, by enabling a wide audience with data access tools and resources to do something with the data. While this path is laid out quite clearly during the project phase, with dedicated platforms to support the initial project goals, it often manifests to be quite challenging in the long term in regard to sustainability. Contributed solutions tend to be more fragmented as originally envisioned, processes and workflows start spanning multiple data locations, multiple applications and multiple services. And consumer data access suddenly becomes a major cost driver (egress costs are still a fundamental part of many cloud business models, but there is hope (opens new window)) making it often necessary to reconsider, if all the data should really be open to everyone or just a subset should be made accessible to certain people.
"The data gravity dance is more important than it was before when you were either distributed or centralised, and now you’re both." Podcast - The Fundamentals Of Data Gravity With Dave McCrory (opens new window)
What may be a reasonable decision for both data providers as well as data consumers at one point may vary over time, making it necessary to change strategy with the potential conclusion to better migrate and move the data. From a data consumer perspective such a data location change can be largely abstracted and won't necessarily be noticed. But for the data provider such a step is usually expensive or even impossible, considering not just data transfer but also the whole ecosystem built around. This makes the overall data location decision crucial from day one.
The competitive landscape in the cloud labelled object storage to be the most flexible and cost effective way to share data, with a largely standardised data access mechanism. By leveraging a data catalogue mechanism on top to search and optionally resolve the linked assets the actual location of the data can be dynamically inferred by applications and services code. Established access token mechanisms (Shared Access Signatures, presigned URLs, etc.) allow access to data with different data governance policies (for a certain time, to a certain group of people, within certain quota limits, etc.), so in federated scenarios with a common mechanism to retrieve an access token for a certain data set this may be fully opaque. The Radiant Earth foundation, widely known in the EO and ML domain with their MLHub for published ML training data, just recently introduced the "Source Cooperative" (opens new window) as a generic data sharing utility, enabling such a federation over different data sets located in different clouds, providing a user-friendly one-stop-shop for data discovery.
The "Source Cooperative" allows data providers to not just seek for outreach through a public listing but also provides all the technical means to govern data access in a unified way. An important point here is that many data providers may want (or need) to retain control over the data, with specific requirements on the data location (e.g. a CDSE hosted bucket). The in the blog post envisioned "Bring your own bucket (BYOB)" approach may enable this scenario by just registering but not actually transferring data. Can this BYOB approach be fully aligned with the general data governance mechanisms and integrated into usage analytics? I hope so, and considering that Source Cooperative is striving to adopt the model of a neutral utility cooperative being fully transparent on usage and costs, I start to see the potential!
Does that help with data gravity in our EO and ML domain full of "open data"? No, I would say even the opposite: By streamlining scalable data access with the conversion of data to new cloud native data formats (COG, Zarr, etc.), indexed in new data catalogues (STAC), accessed through newly created platforms bringing elastic compute close to the data (e.g. Dask/Ray-powered JupyterHubs), we are even fostering data gravity and cause further fragmentation. Does it matter? I also say no, if we succeed to promote the generated "open data" and become more inclusive. And if we take the chance to get a better understanding of the data consumption behaviour and the induced costs with usage analytics collected through a neutral data sharing utility like the "Source Cooperative", to finally be able to make the right data governance decisions: "Shall we deprecate this data set?" "Shall we increase the download quota for this user?" "Shall we still grant access to this user or ask them to pay for their consumption?" "Shall we migrate or even recreate this data set on another cloud?" This will help that budget allocations will be sized right, that the right data will outlive long term and that the right people can access and leverage it. And hopefully that the gravitational pull will start to correlate with the metric of "gained information" instead of just "accessible data".